The implementation of AI has moved from a futuristic dream to a tangible reality in today’s world. This shift in utilizing AI technology is not confined to a single part of the banking industry but spans across the entire spectrum, including the front, middle, and back offices. Whether it involves using sophisticated machine learning algorithms to tackle financial crimes or deploying AI-powered chatbots for customer service, the influence of AI in banking is extensive.
The adoption of AI in banking has streamlined various financial processes, making them more tailored, insightful, and quick. Tasks that used to demand hours of manual work can now be completed within minutes or even seconds with the aid of AI systems.
Despite the banking sector’s historical reliance on technology and data, the emergence of new data-driven AI technologies has expanded the horizons for innovation to unprecedented levels. AI is poised to enhance operational efficiency, drive business growth, differentiate services, address risks and regulatory challenges, and significantly improve customer experiences. The evolution of sophisticated AI systems, which were once seen as expensive and limited to specific applications such as high-frequency trading, is now evolving rapidly. Recent surveys from Deloitte suggest that obstacles to implementing AI, particularly those related to costs and technological constraints, are gradually diminishing, making it more viable for organizations to adopt and integrate AI solutions.
Companies are strategically investing in key areas such as cloud computing, big data platforms, and data applications with modernized architecture that incorporates microservices and event hubs. This strategic approach aims to reduce upfront capital investments usually associated with developing, deploying, and scaling AI solutions in the banking sector.
AI Use Cases in Banking
Let’s delve into some of the most effective applications of artificial intelligence in the banking sector, illustrating how it can improve customer interaction and overall satisfaction.
Personalized Customer Experience
AI-driven chatbots have emerged as a game-changer, offering personalized and immediate responses that cater to individual needs effectively. By leveraging advanced algorithms and continuous learning mechanisms, these chatbots streamline interactions, reduce operational costs, and bolster customer satisfaction and engagement levels. Key benefits include enhancing service quality, increasing engagement through relevant interactions, automating routine inquiries, deepening customer relationships with personalized recommendations, and facilitating real-time support while driving efficiency.
- AI-driven chatbots offer immediate and tailored responses to customer inquiries, improving service quality and satisfaction.
- These chatbots enhance engagement by understanding and addressing individual customer needs and preferences effectively.
- By automating routine inquiries, AI chatbots reduce operational costs and enable human agents to concentrate on more complex tasks.
- Personalized banking recommendations provided by AI chatbots strengthen customer relationships and increase customer retention.
- AI chatbots facilitate real-time issue resolution, leading to reduced wait times for customer support and increased operational efficiency in the banking industry.
Customized Banking Service Recommendations
AI-powered personalized product recommendations revolutionize customer engagement by analyzing transaction history, browsing behavior, and demographics to offer tailored suggestions aligning with customer expectations. This innovative approach not only enhances cross-selling opportunities but also enables customers to make informed financial decisions in sync with their goals and aspirations. Through examples like Erica by Bank of America, AI showcases its prowess in refining service delivery and strengthening customer loyalty.
- AI algorithms analyze customer data for personalized product recommendations.
- Integration of AI recommendation engines in online banking platforms for real-time suggestions.
- Training AI systems on demographic data to enhance the accuracy of offerings.
- Feedback loops refine AI predictive capabilities based on customer responses.
- Compliance with data protection regulations through robust security measures.
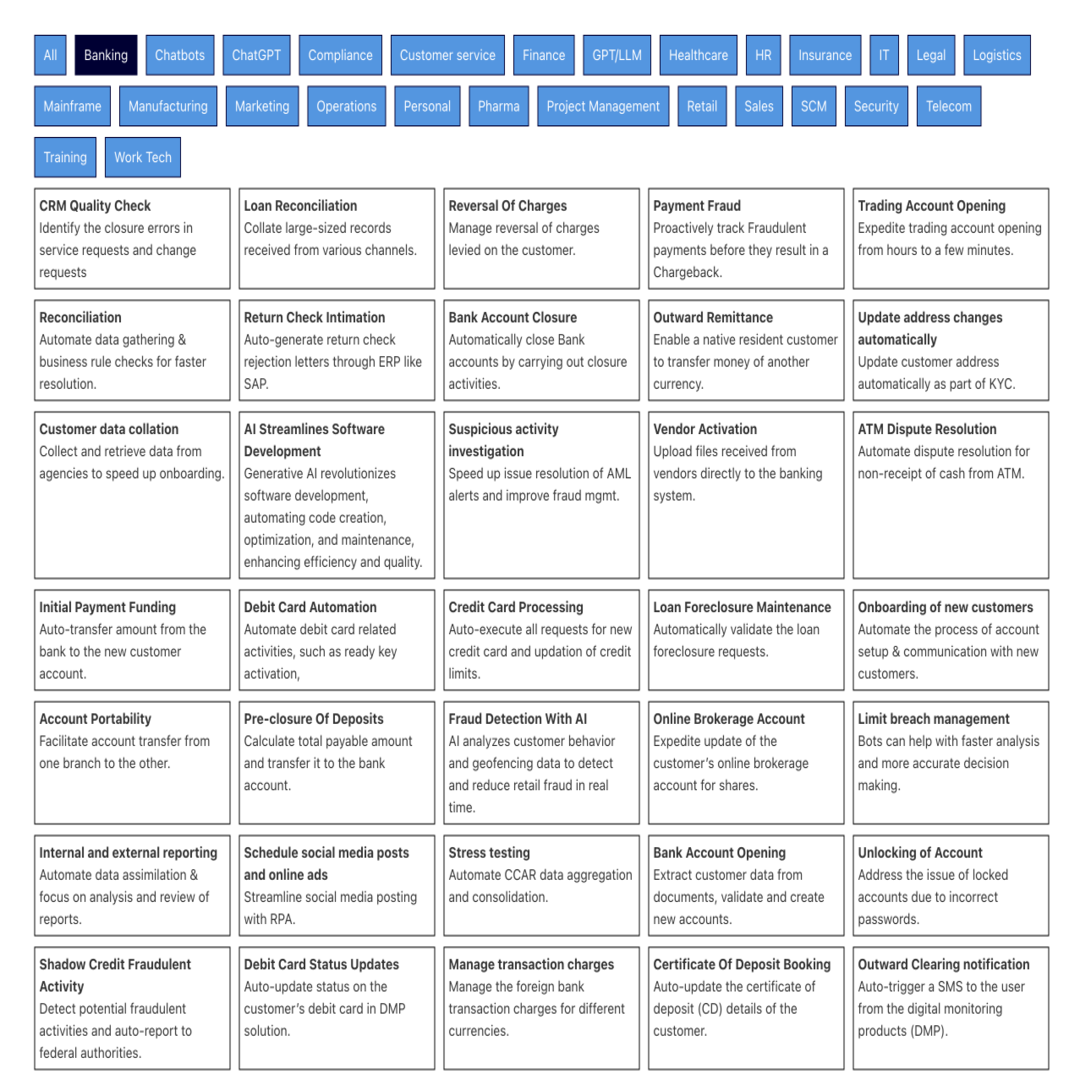
Risk Management
The integration of AI has revolutionized risk management practices, offering unparalleled precision in predicting and mitigating potential threats before they manifest. By leveraging sophisticated algorithms for predictive analytics, financial institutions can harness extensive datasets to detect patterns and forecast risks with unprecedented accuracy. This transformation not only assists in the early identification of financial hazards but also enables the formulation of proactive strategies to mitigate their impact efficiently. The continuous learning capacity of AI from historical data and market trends ensures that risk assessment models evolve, enhancing their accuracy. Automation brought about by AI streamlines the risk management process, facilitating swift identification of potential issues and prompt execution of countermeasures.
- AI integration in credit scoring models enhances default risk prediction accuracy based on historical data, thereby improving loan decision precision.
- AI deployment for fraud detection enables real-time analysis of transaction patterns to identify anomalies indicative of potential fraud, bolstering security measures.
- AI-driven stress testing tools simulate diverse economic scenarios to evaluate bank portfolio resilience against market fluctuations, enhancing risk assessment strategies.
- AI implementation in anti-money laundering (AML) processes scrutinizes transactions efficiently, flagging suspicious activities to combat financial crimes effectively.
- AI adoption in operational risk management predicts system failures and process bottlenecks, facilitating preemptive maintenance actions for seamless operations.
Loan Applications
In the banking sector, the integration of artificial intelligence (AI) and machine learning has revolutionized the loan application process, enabling more accurate credit evaluations and personalized loan products. By analyzing alternative data sources, automating application screenings, monitoring financial health in real time, enhancing security measures, and providing tailored customer service through AI-driven chatbots, banks can expand credit access to underserved populations while reducing default risks and operational costs. These advancements not only streamline loan processing but also foster financial inclusivity, customer satisfaction, and market growth.
- AI and machine learning enhance credit evaluations by analyzing alternative data sources.
- Automation of application screenings speeds up loan processing for quicker fund access.
- Real-time monitoring of financial health allows for dynamic loan term adjustments.
- AI-driven chatbots improve user experience and guide applicants through the loan process.
- Enhanced security measures through natural language processing reduce fraud risks and manual errors.
Cybersecurity and Fraud Detection
AI plays a crucial role in detecting and preventing fraud in the banking and retail sectors by analyzing customer behavior and transaction data in real-time. By leveraging geofencing data and monitoring transaction values, AI systems can proactively identify suspicious activities, such as unauthorized transactions by customers or employees. Regularly updating AI models with new fraud patterns and fostering collaboration between developers and fraud prevention teams ensure continuous refinement of detection models. Educating employees on fraud signs and adjusting the sensitivity of AI systems help strike a balance between minimizing false positives and effectively catching fraudulent activities, ultimately safeguarding operations and brand integrity while reducing financial losses.
- AI in banking and retail sectors enhances real-time fraud detection by analyzing customer behavior and transaction data.
- Geofencing data and order type analysis are utilized to identify fraudulent patterns and secure transactions against unauthorized activities.
- AI systems focus on understanding fraudsters’ intent, such as increasing transaction value per unit, to proactively flag and investigate suspicious transactions.
- Continuous learning and updating of AI models adapt to evolving fraudulent tactics, ensuring robust fraud prevention measures.
- Educating employees on fraud signs and adjusting AI system sensitivity contribute to minimizing false positives and effectively catching fraudulent activities, safeguarding brand integrity and reducing financial losses.
Real-World Examples of AI in Banking
Here are some practical examples of how banking institutions fully leverage AI technology.
- JPMorgan Chase: The research team at JPMorgan Chase has created an advanced early detection system by leveraging AI and deep learning methods to identify malware, trojans, and phishing schemes. According to the researchers, it typically takes 101 days for a trojan to infiltrate corporate networks. This early warning system offers sufficient notice before potential attacks, alerting the bank’s cybersecurity team as cybercriminals gear up to launch malicious email campaigns aimed at infecting the network.
- Capital One: Capital One’s Eno, the intelligent virtual assistant, exemplifies the use of AI in personal banking. In addition to Eno, Capital One utilizes virtual card numbers to enhance security against credit card fraud. Furthermore, the company is developing computational techniques to foster computer creativity and explainability.
Frequently Asked Questions (FAQs) on Generative AI in Banking
1. What is Generative AI and how is it used in the banking sector?
Generative AI refers to models that produce new data based on existing input. In the banking industry, generative AI is used for tasks such as developing personalized financial advice, creating AI chatbots for customer service, and analyzing financial data to identify insights for better decision-making.
2. What are the main use cases of AI in banking and financial services?
AI is increasingly being utilized in banking and financial services for numerous purposes such as fraud detection, customer service automation, risk assessment, personalized recommendations, and streamlining banking operations.
3. How can AI models and machine learning benefit financial institutions?
AI models and machine learning tools can help financial institutions enhance their operations by automating processes, predicting customer behavior, optimizing investments, and improving overall customer experience.
4. What are the potential AI use cases specifically within the banking and finance sector?
Some of the key AI use cases in banking include predictive analytics for loan approvals, chatbots for customer inquiries, anti-money laundering solutions, automated wealth management, and digital payment fraud detection.
5. How can banks leverage generative AI tools to enhance their customer experience?
By integrating generative AI tools, banks can personalize offers for customers, improve fraud detection mechanisms, automate customer interactions, optimize investment strategies, and offer tailored financial advice based on individual needs.