Navigating the Future: Generative AI vs Traditional AI Explained
Artificial Intelligence (AI) is an ever-evolving technology that has the potential to transform every aspect of our lives. AI is already being used in a range of applications, from self-driving cars to virtual assistants like Siri and Alexa. However, as technology evolves, so do the approaches to developing AI. Two of the most popular approaches to AI development are Generative AI and Traditional AI. Generative AI involves creating AI that can create new content, while Traditional AI involves teaching AI to recognize and respond to pre-programmed data. In this blog post, we will take a closer look at both approaches, their pros and cons, and their potential impact on the future of AI. We will also explore how these approaches are being used today and what we can expect from AI in the future. So buckle up and get ready to navigate the future of AI!
Introduction to Generative AI vs Traditional AI
In the world of artificial intelligence, two significant branches have emerged: traditional AI and Generative AI, each with its unique approach and applications. These two approaches offer unique perspectives on how AI can be utilized to solve complex problems and drive innovation.
Traditional AI, also known as rule-based or symbolic AI, relies on predefined rules and structured data to make decisions and perform tasks. It follows a deterministic approach, where the system operates based on logical rules and algorithms. Traditional AI has been instrumental in areas such as machine learning, natural language processing, and computer vision.
On the other hand, Generative AI takes a different approach by focusing on creativity and innovation. This branch of AI, particularly through the use of generative adversarial networks, involves generating new content, such as images, music, and text, based on patterns and trends in existing data. Generative AI uses techniques like neural networks and deep learning to produce novel outputs that mimic human creativity.
As we delve deeper into the realms of Generative AI and Traditional AI, we will explore their applications, benefits, and limitations. By understanding the differences between these two approaches, we can navigate the future of AI and harness its full potential to drive progress and innovation across various industries.
Understanding Traditional AI vs generative AI: How it Works and its limitations
Traditional AI, also known as rule-based AI or symbolic AI, operates based on predefined rules and algorithms. This form of artificial intelligence relies heavily on human-crafted rules and requires explicit instructions for each scenario it encounters. Traditional AI excels in tasks where the rules are well-defined and the data is structured, making it suitable for applications like spam filtering, chess-playing programs, and language translation.
However, traditional AI has its limitations. One significant drawback is its inability to adapt to complex and dynamic environments without constant human intervention to update rules. This restricts its capability to handle unstructured data or tasks with a high degree of variability. Additionally, traditional AI systems may struggle with tasks that involve ambiguity or require nuanced understanding, such as natural language processing or image recognition, which is where generative AI tools can offer significant advantages.
Despite its shortcomings, traditional AI remains valuable in many applications where clear rules and structured data are prevalent, showcasing that the synergy between traditional AI and Generative AI can be very powerful. As technology advances and the need for more adaptive and flexible systems grows, the limitations of traditional AI have paved the way for the development of generative AI.
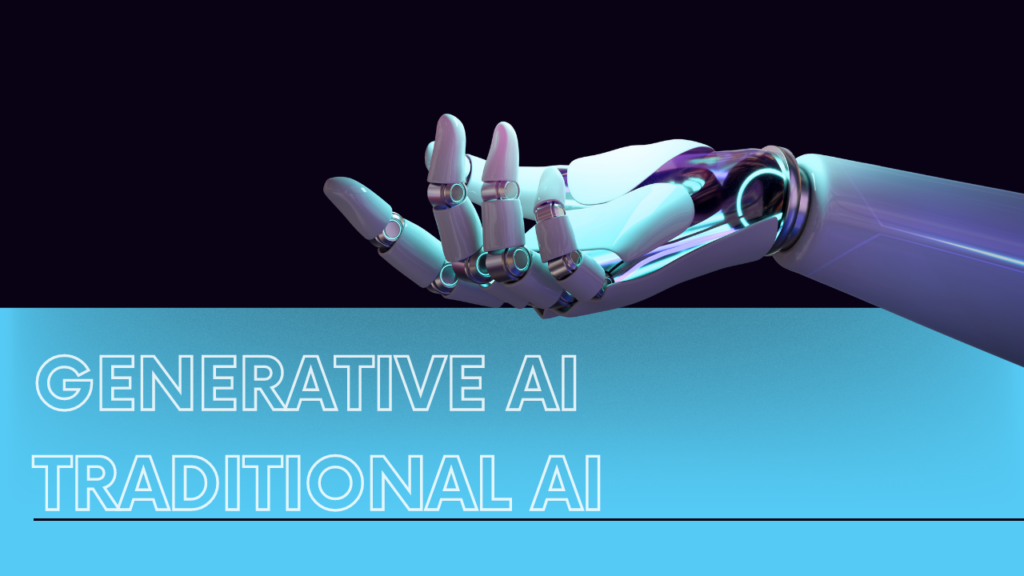
Exploring Generative AI: Definition and applications
Generative AI is a fascinating advancement in artificial intelligence that is revolutionizing various industries. Unlike traditional AI, which is limited to predefined rules and data patterns, generative AI has the ability to create new content, images, or even entire scenarios that have never existed before, marking a significant evolution in the capabilities of AI tools. This innovative technology works by learning patterns and characteristics from a dataset and using that information to generate new, original outputs.
Generative AI finds applications in a wide range of fields, including art, design, music, and even content creation. For instance, in the field of art, generative AI can be used to create unique and visually stunning artworks by analyzing existing masterpieces and generating new pieces inspired by those styles. In the design industry, generative AI can assist designers in creating innovative and personalized products tailored to individual preferences.
Moreover, generative AI is also making waves in the music industry by composing original pieces based on existing music styles and genres. This technology enables musicians and composers to explore new creative avenues and push the boundaries of traditional music composition.
Overall, generative AI offers endless possibilities for innovation and creativity, making it a powerful tool for shaping the future of various industries. By understanding its definition and applications, businesses and individuals can leverage generative AI to unlock new opportunities and stay ahead in the rapidly evolving digital landscape.
Key differences between Generative AI and Traditional AI
Generative AI and Traditional AI represent two distinct approaches within the realm of artificial intelligence, each with its own set of characteristics and applications. Understanding the key differences between the two can shed light on their respective strengths and limitations.
Traditional AI, also known as “classic” or “narrow” AI, operates within predefined parameters and is designed to perform specific tasks or solve particular problems. This type of AI relies heavily on structured data and follows a rule-based approach to decision-making. Traditional AI excels in tasks such as image recognition, natural language processing, and predictive analytics, where the input data is well-defined and the desired output is clear.
On the other hand, Generative AI represents a more advanced form of artificial intelligence that is capable of creating new content or data that has not been explicitly programmed. This type of AI leverages techniques such as neural networks and deep learning to generate output that is original and creative. Generative AI is used in applications like image generation, text-to-image synthesis, and music composition, where the goal is to produce novel and diverse outputs.
One key distinction between Generative AI and Traditional AI lies in their approach to problem-solving. While Traditional AI focuses on optimizing performance within a defined domain, Generative AI seeks to explore and innovate beyond existing boundaries. This difference in mindset enables Generative AI to push the boundaries of creativity and generate new possibilities that were previously unexplored.
In summary, the key differences between Generative AI vs Traditional AI lie in their approach to problem-solving, their reliance on structured data versus creative output generation, and their respective capabilities in defined versus open-ended tasks. By understanding these distinctions, organizations can better navigate the evolving landscape of artificial intelligence and harness the unique strengths of each approach to drive innovation and progress.
Use cases of Generative AI in various industries
Generative AI, with its ability to create new content and generate realistic output, has opened up a world of possibilities across various industries. Let’s delve into some intriguing and innovative use cases of Generative AI:
1. Art and Design: Generative AI is revolutionizing the creative industry by assisting artists and designers in generating unique artwork, designs, and digital content. From creating intricate patterns to generating new art styles, Generative AI tools are pushing the boundaries of creativity.
2. Healthcare: In the healthcare sector, Generative AI is being utilized to analyze medical data, assist in disease diagnosis, and even predict patient outcomes. By leveraging Generative AI, healthcare professionals can enhance treatment plans and improve patient care.
3. Finance: Generative AI is transforming the financial sector by optimizing trading strategies, generating financial reports, and detecting fraudulent activities. With the ability to analyze vast amounts of data and generate insights, Generative AI is reshaping the way financial decisions are made.
4. Gaming: Generative AI is powering the next generation of immersive gaming experiences by creating dynamic environments, personalized game scenarios, and intelligent non-player characters. Gamers can now enjoy more interactive and engaging gameplay thanks to Generative AI algorithms.
5. Marketing and Advertising: In the realm of marketing and advertising, Generative AI is helping businesses create personalized content, optimize ad campaigns, and predict consumer behavior. By using generative AI to generate compelling and targeted marketing materials, companies can enhance their brand presence and attract more customers.
These are just a few examples of how Generative AI is making a significant impact across diverse industries. As technology continues to advance, the potential applications of Generative AI are boundless, offering endless opportunities for innovation and growth.
Advantages and challenges of Generative AI
Generative AI is a cutting-edge technology that has been making waves in various industries. One of the key advantages of Generative AI is its ability to create new and original content, whether it’s in the form of images, text, music, or even videos. This opens up a world of possibilities for businesses looking to innovate and differentiate themselves in a competitive market.
Another advantage of Generative AI is its ability to understand and interpret complex data sets, leading to more accurate predictions and insights. This can be particularly valuable in fields such as healthcare, finance, and marketing, where data-driven decisions are crucial.
However, with great power comes great responsibility, and Generative AI also presents its own set of challenges. One of the main concerns is the potential for misuse, such as generating fake news or deepfakes that can spread misinformation and harm individuals or organizations.
Moreover, the ethical implications of Generative AI raise questions about privacy, security, and bias in the algorithms used to generate content. As businesses and developers navigate the landscape of Generative AI, it is essential to prioritize transparency, accountability, and ethical considerations to harness its full potential while mitigating risks.
Real-world examples of Generative AI applications
Generative AI, with its ability to create new content and generate diverse outputs, is revolutionizing various industries. Let’s delve into some real-world examples of Generative AI applications that showcase its potential:
1. Art and Design: Generative AI is being used to create unique pieces of art, designs, and animations. Artists and designers are leveraging generative AI tools to generate novel and creative visuals that push the boundaries of traditional art forms.
2. Content Creation: In the media and entertainment industry, Generative AI is being utilized to generate personalized content such as articles, stories, and even music. This technology can analyze user preferences and behavior to create tailored content experiences.
3. Virtual Assistants and Chatbots: Generative AI powers advanced virtual assistants and chatbots that can engage in natural language conversations with users. These AI-driven assistants are capable of generating human-like responses and understanding complex queries.
4. Healthcare: Generative AI is making significant strides in healthcare by assisting in medical image analysis, drug discovery, and personalized treatment plans. This technology can generate insights from vast amounts of data to improve patient care and outcomes.
5. Gaming and Simulation: In the gaming industry, Generative AI is used to create dynamic environments, intelligent non-player characters (NPCs), and immersive gameplay experiences. This technology can generate game elements in real time, enhancing player engagement.
These examples highlight the diverse applications of Generative AI across various sectors, showcasing its ability to drive innovation, creativity, and efficiency in the digital age. As the technology continues to evolve, we can expect to see even more groundbreaking applications that redefine how we interact with AI in our daily lives.
Combining Generative AI and Traditional AI for enhanced outcomes
To truly harness the power of artificial intelligence, combining generative AI and traditional AI techniques can lead to groundbreaking outcomes. Generative AI focuses on creativity and imagination, enabling machines to generate unique content such as images, text, and even music. On the other hand, traditional AI excels in tasks like pattern recognition and data analysis.
By integrating these two approaches, businesses can achieve enhanced results across various applications. For instance, in the field of image recognition, traditional AI can accurately identify objects while generative AI can create realistic images based on incomplete data. This synergy can revolutionize industries like healthcare, finance, and entertainment by improving accuracy, efficiency, and innovation.
Moreover, the combination of generative and traditional AI can lead to the development of highly personalized products and services, tailored to individual preferences and needs. This hybrid approach paves the way for a new era of AI applications that are not only intelligent and efficient but also creative and adaptive to complex challenges.
In conclusion, embracing the synergy between generative AI and traditional AI holds immense potential for businesses seeking to navigate the future of artificial intelligence. By leveraging the strengths of both approaches, organizations can unlock unprecedented opportunities and drive transformative outcomes in the ever-evolving landscape of AI technology.
Ethical considerations and implications of Generative AI
Ethical considerations surrounding Generative AI are paramount in the discussion of its implementation and impact on society. As Generative AI becomes more advanced, there are concerns about its potential misuse, such as deepfake technology being used to create deceptive content or manipulate public opinion.
One of the key ethical implications of Generative AI, especially in applications like chatgpt, is the issue of consent and privacy. With the ability to generate highly realistic fake images, videos, and audio, there is a risk of individuals being misrepresented or having their likeness used without permission. This raises questions about the rights of individuals to control their own digital identities and the need for robust regulations to protect against misuse.
Moreover, there are concerns about the potential for Generative AI to perpetuate biases and stereotypes present in the data it is trained on. If the training data is biased, the AI models generated from it may also exhibit bias, leading to discriminatory outcomes in decision-making processes. Addressing these biases requires careful consideration and proactive measures to ensure fairness and equity in AI systems.
In navigating the future of Generative AI, it is crucial for stakeholders to engage in thoughtful discussions about the ethical implications of this technology and work towards developing frameworks and guidelines that prioritize the well-being and rights of individuals. By approaching Generative AI with a strong ethical foundation, we can harness its potential for positive innovation while mitigating risks and safeguarding against harm, ensuring that AI continues to evolve in a responsible manner.
The future of AI: Potential advancements and trends in Generative AI
Generative AI, with its ability to create new content rather than just recognize patterns in existing data, holds immense potential for shaping the future of artificial intelligence. As we look ahead, the advancements and trends in Generative AI are set to revolutionize various industries and applications.
One of the key areas where Generative AI is expected to make significant strides is in the field of creative content generation. From art and music to literature and design, Generative AI algorithms are already being used to produce original works that push the boundaries of human creativity. This opens up new possibilities for artists, writers, and creators to explore fresh ideas and push the limits of innovation.
Moreover, Generative AI is poised to transform the way we interact with technology through the development of more personalized and adaptive user experiences. By leveraging Generative AI models, companies can tailor their products and services to individual preferences, delivering hyper-personalized recommendations and solutions that enhance customer satisfaction and engagement.
In addition, the advancements in Generative AI are expected to drive breakthroughs in fields such as healthcare, finance, and scientific research. By harnessing the power of Generative AI, researchers and professionals can accelerate the pace of discovery, uncovering new insights and solutions to complex problems that were previously unattainable.
Overall, the future of AI lies in the hands of Generative AI, with its potential to unlock unprecedented capabilities and drive innovation across diverse sectors. By staying abreast of the latest advancements and trends in Generative AI, we can navigate the future with a deeper understanding of its transformative impact on society and technology.
In this blog post, we’ve delved into the exciting realm of artificial intelligence, exploring the differences between generative AI and traditional AI. As technology continues to evolve at a rapid pace, it’s crucial to understand these distinctions and their implications for the future. By grasping the potential of generative AI and its ability to create new content, we can better navigate the ever-changing landscape of AI technology. We hope this post has provided valuable insights and sparked your curiosity about the limitless possibilities that lie ahead in the realm of artificial intelligence. Stay informed, stay curious, and embrace the future with open arms.
Generative AI vs Traditional AI FAQ
Q1.What is the difference between Generative AI and Traditional AI?
Ans: While Traditional AI focuses on using predefined logic and rules to generate specific outputs, Generative AI has the potential to create new, original content on its own, often without explicit programming or rules.
Q2.How do Generative AI models differ from traditional AI models?
Ans: Generative AI models are designed to learn the underlying patterns of data and generate new content, such as images, text, or audio, autonomously. On the other hand, Traditional AI models are typically designed for specific tasks based on predefined rules or algorithms.
Q3. What are some examples of Generative AI applications?
Ans: Examples of Generative AI applications include creating realistic images, generating human-like text, composing music, and even deepfake technology.
Q4.How can Generative AI be used in real-world scenarios?
Ans: Generative AI can be utilized in various fields such as content creation, design automation, personalized recommendations, and even in generating synthetic data for training AI models.
Q5. What are the potential benefits of using Generative AI over Traditional AI?
Ans: Generative AI has the potential to provide more creative and diverse outputs compared to Traditional AI, which is often limited by explicit rules and predefined tasks.
Q6. Are there specific tools or technologies associated with Generative AI?
Ans: Generative AI tools may include platforms like ChatGPT and techniques like Generative Adversarial Networks (GANs) which are commonly used for generating realistic content.
Q7. How does Generative AI differ from Traditional AI in terms of applications?
Ans: Generative AI can be used for creating novel content and generating variations, while Traditional AI typically focuses on predictive and task-specific applications.