Embarking on the journey to use generative AI to create new strategies in your business can unlock new avenues for innovation and efficient introduction to generative AI: A step-by-step guide. This comprehensive guide is designed to navigate you through the essentials of getting started with generative AI to enhance your understanding and application of this transformative technology.
Generative artificial intelligence, or generative AI, is a type of generative AI technology that can create new content, strategies, or ideas through machine learning algorithms. By harnessing the power of generative AI, businesses can speed up the process of innovation and bring new products and services to market faster than ever before.
By using generative AI in your business, you can unlock new opportunities for innovation and efficiency, showcasing the practical generative AI use in various industries. By following the guidelines outlined in this self-study guide, you can successfully navigate the world of generative AI and start reaping the benefits of this transformative technology.
Introduction
Generative AI in 2024 presents a revolutionary approach to how businesses approach innovation, content creation, and problem-solving by offering advanced models for text generation and creative applications. By harnessing the power of artificial intelligence to generate new data and ideas, companies can achieve unprecedented levels of creativity and efficiency. Generative AI works by training algorithms on large datasets to learn patterns and understand complex relationships. This allows the AI to mimic human creativity and use generative AI to create new ideas, solutions, and content that can be used to improve products, services, and operations.
One of the key benefits of generative AI is its ability to produce a wide range of diverse and novel ideas that may not have been thought of by human creators through advanced techniques like natural language processing. This can help businesses explore new opportunities, discover potential risks, and develop innovative solutions to complex problems, all through the lens of generative AI’s impact.
For example, Netflix improved personalized recommendations, enhancing viewer engagement and content discovery. Airbnb utilized AI for listing descriptions, making them more appealing and accurate. These examples demonstrate generative AI’s power in tailoring user experiences and streamlining content creation, showcasing significant efficiency and engagement improvements.
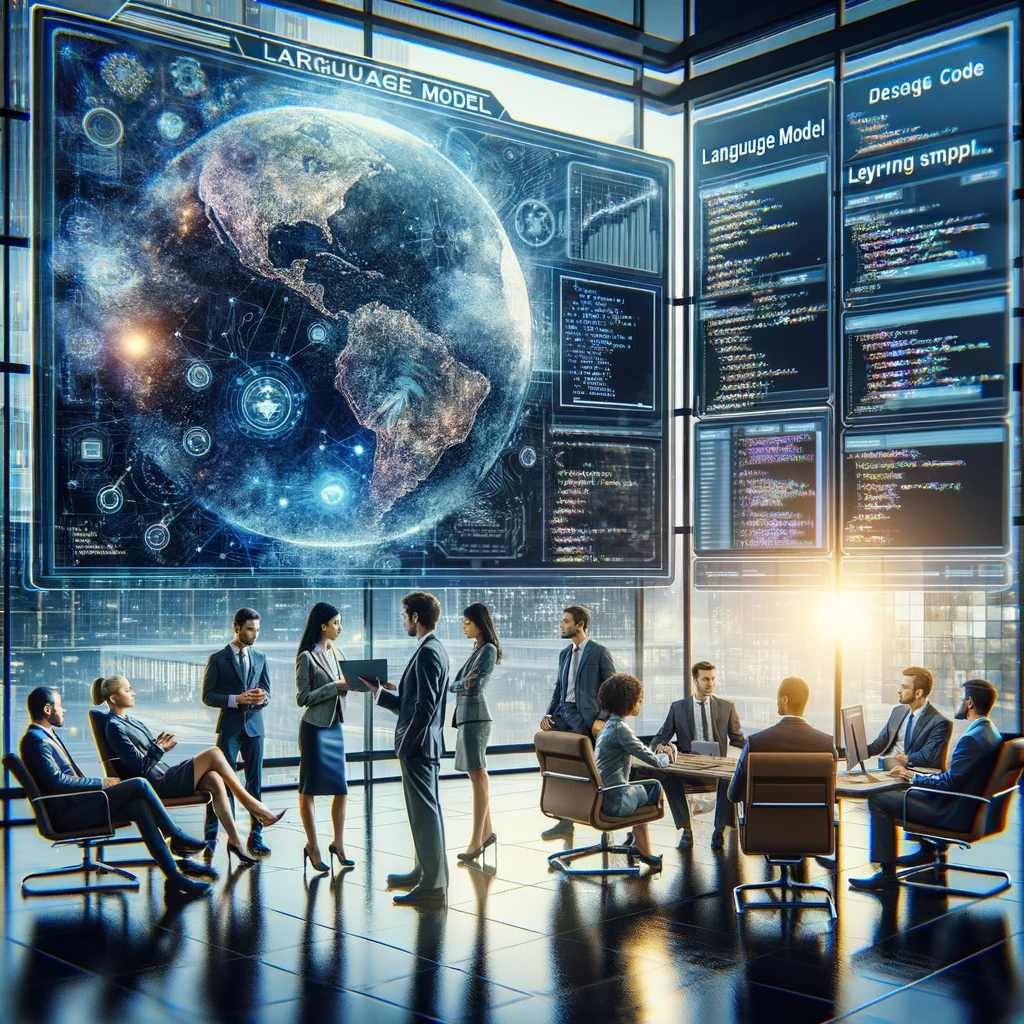
Overall, generative AI presents exciting possibilities for transforming the way businesses innovate, create value, and utilize AI tools in their operations. By leveraging the power of artificial intelligence to generate new ideas and solutions, companies can stay ahead of the competition and drive growth in today’s fast-paced digital economy.
Section 1: Understanding Generative AI
Large Language Model
Large language models are at the forefront of generative AI, enabling machines to understand and produce human-like text. These models, trained on vast amounts of text data, can generate coherent and contextually relevant content on demand. One of the most well-known language models like OpenAI’s GPT-3, which has 175 billion parameters and has been trained on a wide range of internet text, encompasses natural language processing and text generation abilities. These models have a wide range of applications, from chatbots and virtual assistants to content generation and machine translation.
Large language models can understand and generate natural language text, allowing them to answer questions, generate creative writing, and even engage in meaningful conversations with users. They are trained to recognize patterns in language and can generate text that is grammatically correct and contextually relevant.
However, there are concerns about the ethical implications of using large language models, particularly in terms of bias and misinformation. Researchers are working on ways to mitigate these risks and ensure that language models are used responsibly and ethically.
Overall, large language models have the potential to revolutionize how we interact with machines and how we use generative AI to create and consume content, showcasing the exciting possibilities of generative AI. With further advancements in AI technology, we can expect to see even more sophisticated and intelligent language models in the future, especially in the realm of generative AI for beginners and experts alike.
Generative AI Models
A generative AI model learns from data to generate new content that mimics the original input, emphasizing the impact of generative AI across various fields. These models are used for a range of tasks, including creating realistic images, composing music, writing coherent text passages, and other forms of content creation through generative AI tools. Generative AI models work by learning the underlying patterns and structures of the input data and then using that knowledge to generate new content that is similar to the original data. These models can be trained on a wide variety of data sources, such as images, text, audio, and more, to produce new content that is indistinguishable from the originals.
One of the most well-known applications of generative AI is in the field of image generation, where models like Generative Adversarial Networks (GANs) can create highly realistic images that are often indistinguishable from photos taken by a human. These models have been used in a variety of applications, such as creating fake faces for use in advertising or generating new artwork.
In the realm of music, generative AI models can be used to compose new pieces of music based on existing compositions or to create entirely new musical styles. These models can analyze musical patterns and structures to generate new melodies, harmonies, and rhythms that sound like they were composed by a human musician.
Generative AI models can also be used to generate text passages, such as stories, poems, or articles. By analyzing large amounts of text data, these models can learn how to generate new text that is coherent, grammatically correct, and stylistically consistent with the input data, demonstrating generative AI’s capability for beginners and advanced users.
Overall, generative AI models have a wide range of applications in content creation and can be used to generate new content in a variety of forms. As the field of AI continues to advance, we can expect to see even more impressive applications of generative AI in the future.
Machine Learning vs. Gen AI
Machine learning is a subset of AI focused on algorithms that learn from data to make predictions or decisions, laying the groundwork for the generative AI journey. Generative AI, on the other hand, uses machine learning to create new data that resembles the training set, pushing the boundaries of AI’s creative capabilities and hinting at the future of generative AI. Generative AI is able to create new content, such as images, music, or text, that is similar to the examples it has learned from. This can be used for tasks such as generating realistic images of people who don’t exist, creating new music compositions, or even writing stories in the style of a particular author.
One of the popular techniques used in generative AI is called Generative Adversarial Networks (GANs), where two neural networks are trained simultaneously – one to generate the content and the other to discriminate between real and generated examples. This competition between the two networks pushes the generator to create more realistic content.
Generative AI has a wide range of applications, from generating artwork and music to enhancing data for training machine learning models. It has the potential to revolutionize creative industries and help researchers in various fields generate new ideas and solutions. However, there are also ethical concerns surrounding the use of generative AI, such as the potential for misuse in creating fake news or deepfakes. As the technology continues to advance, it will be important to consider these implications and ensure that it is used responsibly.
Applications of Generative AI
Generative AI applications span various domains, from text and image generation to music and video creation, illustrating the capabilities of generative AI. These technologies can automate content creation, personalize user experiences, and generate innovative solutions to complex problems. Some common examples of generative AI applications include:
1. Text Generation: AI can be used to generate news articles, product reviews, social media posts, and even poetry. Tools like OpenAI’s GPT-3 can generate human-like text based on a given prompt.
2. Image Generation: AI can create realistic images from scratch or modify existing images, a testament to how AI can generate new visual content. This can be used for generating stock photos, creating digital art, or enhancing photographs.
3. Music Generation: AI can compose original music in various styles and genres. Companies like Amper Music and Jukedeck offer AI-powered music composition tools for creating background music for videos, podcasts, and other content, illustrating the impact of generative AI in creative industries.
4. Video Creation: AI can automate the process of video editing, generate video captions, and even create deepfake videos. Tools like Lumen5 and Magisto use AI algorithms to help users create engaging video content.
Introduction to generative AI can help kickstart your learning journey in this innovative field. Personalized User Experiences: AI can analyze user data and behavior to generate personalized recommendations, product suggestions, and customized content. This can improve user engagement and drive conversions for businesses.
6. Creative Problem Solving: AI can be used to generate creative solutions to complex problems by exploring a wide range of possibilities and coming up with novel ideas. This can be useful in fields like design, engineering, and research.
Overall, generative AI applications have the potential to revolutionize content creation, user experiences, and problem-solving processes across various industries. As these technologies continue to evolve, we can expect to see more innovative uses of generative AI in the future.
Section 2: Prerequisites for Getting Started with Gen aI
Grasping the Basics of AI
The journey begins with understanding the fundamentals of AI. Despite the technical jargon, the essence of AI is to augment human capabilities and streamline operations. AI can be categorized into five archetypes based on the type of data they process:
- Supervised Machine Learning
- Natural Language Processing,
- Audio and Voice Recognition
- Computer Vision
- Generative AI.
Each archetype opens a different door to enhance your business processes, from automating customer service through chatbots to generating new content or analyzing customer data for insights. Spending a few hours to grasp these concepts can significantly demystify AI for you.
Understanding the Basics of Generative AI
At the heart of generative AI models lies the ability to create new, previously unseen data based on the patterns and information they’ve learned. This includes everything from text generation to image generation, making them invaluable for a wide range of applications. For those unfamiliar, a generative AI model can analyze extensive datasets to generate output that mimics the original data, enabling capabilities such as creating realistic images or composing text. Machine learning and natural language processing are subsets of artificial intelligence that play crucial roles in developing these models, including large language models known for their ability to understand and produce human-like text.
One of the key aspects of working with and getting started with Generative AI is Prompt Engineering.
Prompt Engineering
Prompt engineering involves designing and refining inputs to elicit desired outputs from AI models. It’s a crucial skill for leveraging generative AI tools, requiring a deep understanding of how different prompts can influence the model’s responses. Prompt engineering is a nuanced process that involves carefully crafting the wording, structure, and context of prompts to guide the AI model towards producing the desired outputs. This skill is essential when working with generative AI tools, such as language models or image generators, on an ai platform, as the quality and relevance of their outputs heavily depend on the inputs provided.
One key aspect of prompt engineering is understanding the characteristics and capabilities of the AI model being used. Different models have different strengths and weaknesses, and knowing how to tailor prompts to leverage these attributes is essential for achieving the best results. For example, some models may excel at generating creative writing based on open-ended prompts, while others may perform better with more specific and structured inputs.
Another important factor in prompt engineering is considering the intended goal or outcome of the AI-generated content. Whether it’s writing a story, composing music, or generating artwork, the prompts need to be designed in a way that guides the model toward producing outputs that align with the desired aesthetic, tone, or theme.
Furthermore, prompt engineering requires a deep understanding of how language, context, and cultural nuances can influence the model’s responses, highlighting the impact of generative AI on the AI journey. Choosing the right words, framing the prompt in a relevant context, and providing additional context or constraints can significantly impact the quality and coherence of the AI-generated outputs.
Overall, prompt engineering is a crucial skill for effectively harnessing the power of generative AI tools, essential for navigating the future landscape of AI in 2024. By mastering the art of crafting and refining inputs, engineers can unlock the full potential of AI models and create compelling, meaningful content that meets their specific needs and objectives.
Section 3: Choosing the Right Model and Framework
Selecting the appropriate model and framework is critical to the success of your generative AI projects. Factors to consider include the model’s complexity, the specificity of the task, the computational resources available, and the model’s ability in text generation. Some common models and frameworks used in generative AI projects include:
1. OpenAI’s GPT (Generative Pre-trained Transformer) models: These models have been widely used in natural language processing tasks and are known for their ability to generate coherent and contextually relevant text. They are often used for tasks such as language translation, text summarization, and content generation.
2. LSTM (Long Short-Term Memory) networks: These are recurrent neural networks that are well-suited for sequential data like text. They have been used in tasks such as text generation, speech recognition, and sentiment analysis.
3. Transformer models: These models, particularly the BERT (Bidirectional Encoder Representations from Transformers) model, have shown great results in natural language processing tasks, marking a significant development in the field of generative AI. They are known for their ability to understand the context of words in a sentence and generate text accordingly.
4. Tensorflow and PyTorch: These are popular deep learning frameworks that provide a wide range of tools and resources for building and training generative AI models. They are known for their flexibility, scalability, and ease of use.
When selecting a model and framework for your generative AI project, consider the specific requirements and constraints of your task. For example, if you are working with a large dataset and have access to powerful computational resources, you may opt for a complex model like GPT-3. On the other hand, if you are working with a smaller dataset and limited resources, you may choose a simpler model like an LSTM network.
It is also important to consider the model’s ability in text generation. Some models may excel in generating coherent and contextually relevant text, while others may struggle with generating diverse and creative outputs. Evaluate the strengths and weaknesses of different models and frameworks before making a decision.
Section 4: Identifying Use Cases for Generative AI
Get Inspired by Real-world Applications
Exploring real-world applications of generative AI can provide valuable insights into its potential impact and inspire innovative use cases within your own business.
Some potential real-world applications of generative AI include:
1. Content creation: Generative AI can also be used to generate high-quality content such as articles, blog posts, or social media posts. This can help businesses automate content creation processes and produce personalized content for their audience.
2. Design and creativity: Generative AI can be used to create original designs, artwork, or music. This can be particularly useful in industries such as fashion, graphic design, or music production, where generative AI can help spur innovation.
3. Personalized recommendations: Generative AI can be used to analyze customer data and generate personalized recommendations for products or services. This can help businesses improve customer engagement and increase sales.
4. Fraud detection: Generative AI can be used to analyze patterns in financial transactions and detect potential cases of fraud, showcasing how AI in 2024 will continue to enhance security measures. This can help businesses reduce financial losses and protect their customers from fraudulent activities.
5. Virtual assistants: Generative AI can be used to create chatbots or virtual assistants that can interact with customers and provide personalized support. This can help businesses improve customer service and streamline communication processes.
By exploring these and other real-world applications of generative AI, businesses can gain a better understanding of the technology’s capabilities and potential impact. This can inspire innovative use cases within their organization and help them leverage generative AI to drive business growth and success. Workshops
Identifying Use Cases for Generative AI
The first step in leveraging generative AI tools is to identify specific use cases within your business where they can have the most impact. Use cases for generative AI span a broad spectrum, from automating content creation to enhancing user interactions with natural language processing. By pinpointing where generative models can solve existing challenges or unlock new opportunities, businesses can tailor their AI strategy to address specific needs. This might involve using generative AI to create unique customer experiences or to generate high-quality content efficiently.
Organizing workshops and hackathons can offer hands-on experience with generative AI technologies, helping to identify practical use cases and foster a deeper understanding of these systems.
Section 5: Aligning AI Capabilities with Business Needs
With a clear understanding of your business challenges, the next step is to align AI capabilities with these challenges. This doesn’t mean looking for a one-size-fits-all tool but rather identifying how AI can complement and enhance specific areas of your operations. For example, if customer engagement is a challenge, Natural Language Processing technologies could be a game-changer for your customer service department. This stage is about matching problems with AI solutions in a way that is coherent and strategic.
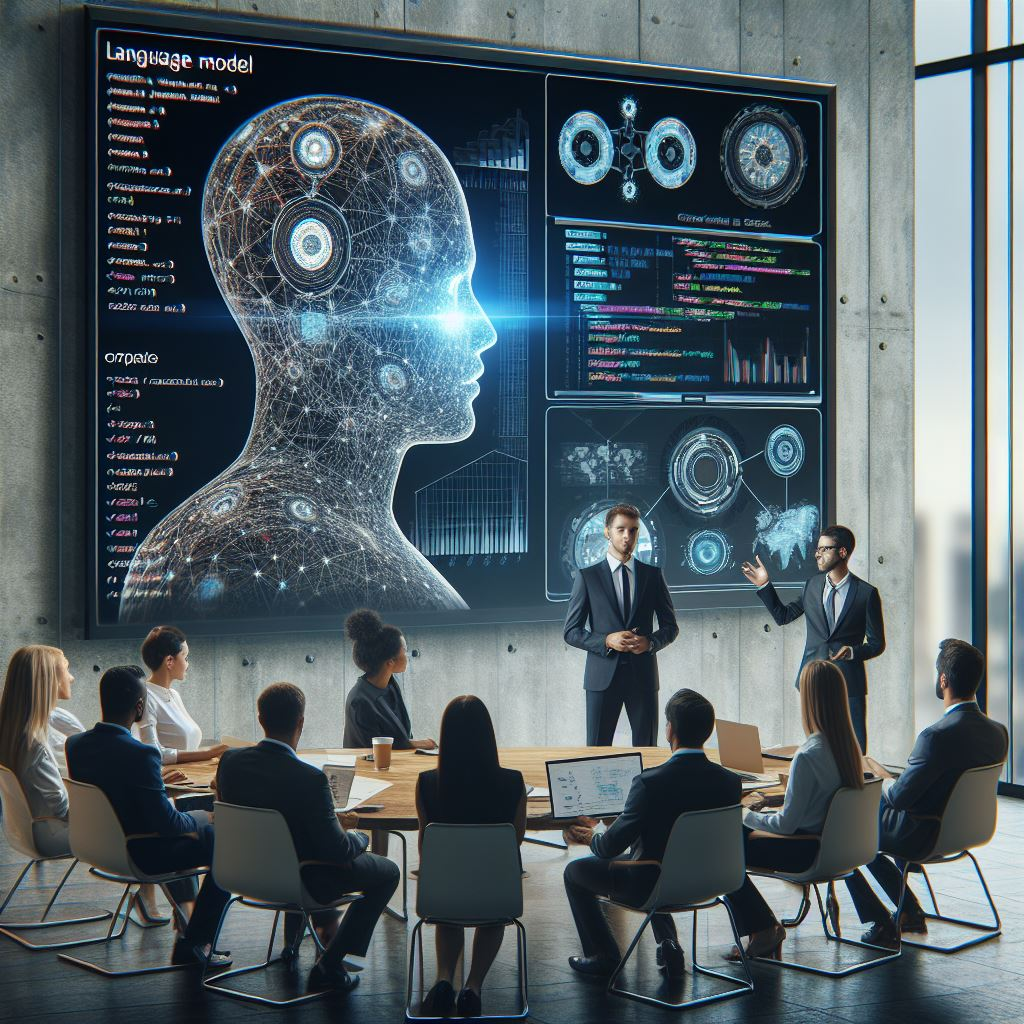
Identifying where generative AI can address specific business challenges or enhance operations is key to leveraging its potential. This requires a clear understanding of both the technology’s capabilities including natural language processing and the unique needs of your business. Here are some specific business challenges where generative AI can be beneficial:
1. Customer service: Generative AI can be used to create chatbots that can interact with customers in a natural language, providing support and assistance 24/7.
2. Content creation: Generative AI can be used to automatically generate content such as product descriptions, blog posts, and social media posts, saving time and resources for content creators.
3. Personalization: Generative AI can analyze large amounts of data to create personalized recommendations for customers, such as product suggestions or content recommendations.
4. Creative design: Generative AI can be used to create unique and innovative designs for products, marketing materials, and advertisements.
5. Fraud detection: Generative AI can be used to analyze patterns in data to detect fraudulent activity, helping to protect businesses from financial losses.
By understanding how generative AI can address these specific challenges, businesses can develop strategies to incorporate this technology into their operations and stay ahead of the competition.
Section 6: Evaluating and Prioritizing AI Applications
Evaluating Use Cases
Once you’ve identified potential AI applications, it’s time to evaluate specific use cases. This involves a detailed analysis of how AI can be applied to address the challenges identified earlier, assessing the feasibility and potential ROI of each application. It’s essential to keep this analysis focused and realistic, considering both the technical and financial aspects of implementing AI solutions. The goal here is to prioritize use cases that offer tangible benefits and are achievable within your resources.
Evaluating potential AI applications involves assessing their feasibility, potential impact, and alignment with business objectives. Prioritizing use cases ensures that resources are allocated to the most promising projects, especially those that can benefit from generative AI and learning models, paving the way for the future of generative AI. To evaluate potential AI applications, businesses should consider the following factors:
1. Feasibility: Assess the technical feasibility of the AI application, including data availability, infrastructure requirements, and potential challenges in implementation.
2. Potential impact: Evaluate the potential benefits of the AI application, such as increased efficiency, cost savings, improved decision-making, and enhanced customer experience.
3. Business objectives: Align the AI application with the overall business objectives and strategic goals of the organization to ensure that it contributes to long-term success.
4. Prioritization: Prioritize AI applications based on their feasibility, potential impact, and alignment with business objectives to allocate resources effectively and focus on the most promising projects.
5. Generative AI and learning models: Consider the use of generative AI and learning models to create AI applications that can generate new content, images, or designs, leading to innovative solutions and competitive advantages.
By carefully evaluating potential AI applications and prioritizing use cases, businesses can effectively leverage AI technology to drive growth, innovation, and success in today’s competitive marketplace.
Section 7: Prototyping and Iterating with Generative AI
After evaluating your use cases, prioritize them based on their impact and feasibility. Start with projects that promise quick wins and require minimal upfront investment. This approach allows for tangible results that can build momentum and support for more complex AI initiatives. Prototyping these solutions within a short timeframe and with a clear budget cap ensures that you can test and iterate without significant risk.
Prototyping allows for the testing and refinement of generative AI applications before full-scale implementation, showcasing the practical use of AI tools in developing solutions. Iteration is crucial, as continuous feedback and adjustment can significantly improve the performance and relevance of AI systems, particularly in the AI development process. By prototyping AI applications, developers can identify potential flaws, gaps in functionality, and areas for improvement early in the development process. This iterative approach allows for rapid iteration and experimentation, enabling developers to refine the AI model, optimize algorithms, and enhance user experience.
Prototyping also provides a tangible representation of the AI application, making it easier for stakeholders to understand the concept and functionality of the system. This helps in garnering feedback from users, domain experts, and other stakeholders, which can be invaluable in shaping the final product with the capabilities of generative AI. Additionally, prototyping allows for better estimation of resources and timelines for full-scale implementation, reducing the risks and uncertainties associated with AI projects.
Overall, prototyping plays a crucial role in the development of generative AI applications by facilitating iterative testing, refinement, and validation of the technology. It enables developers, including AI experts, to address potential issues early on, optimize performance, and ensure that the AI system meets the desired requirements and objectives. In this way, prototyping helps in bridging the gap between theory and practice, demonstrating the practical utility of AI tools in solving real-world problems.
Section 8: Ethical Considerations
Ethical considerations are paramount when implementing generative AI. Issues such as data privacy, bias, and the potential for misuse must be addressed to ensure responsible and beneficial use of the technology. When implementing generative AI, it is important to consider the ethical implications of the technology to ensure it aligns with the principles of responsible AI. One major concern around data privacy is heightened in the field of generative AI. Generative AI algorithms require large amounts of data to be trained on, and this data may contain sensitive information about individuals. It is important to ensure that this data is handled securely and that privacy regulations are adhered to.
Another ethical consideration is bias. Generative AI algorithms can inadvertently perpetuate biases present in the data they are trained on. This can lead to unfair or discriminatory outcomes, particularly in applications like hiring or loan approvals. It is important to carefully evaluate the data used to train the algorithm and to actively work to mitigate bias in the system.
Furthermore, there is a concern about the potential for misuse of generative AI technology. For example, deepfake videos created using generative AI can be used to spread misinformation or manipulate individuals. Careful oversight and regulation are needed to prevent such misuse and ensure that generative AI is used in ways that benefit society.
Overall, it is essential to approach the implementation of generative AI with a strong ethical framework in mind. This includes considerations around data privacy, bias, and potential misuse, as well as ongoing monitoring and evaluation to ensure that the technology is being used responsibly and ethically.
Conclusion
Integrating generative AI into your business opens a world of possibilities for innovation, efficiency, and customer engagement. By understanding the fundamentals, identifying use cases, and aligning AI capabilities with business needs, you can navigate the complexities of AI integration and leverage its full potential for your business. Remember, the journey to successfully implementing generative AI is iterative, requiring continuous learning and adaptation to the evolving landscape of AI technologies. Here are some key steps to consider when integrating generative AI into your business:
1. Educate yourself and your team on the basics of generative AI and its applications in your industry. This will help you understand the capabilities and limitations of the technology and how it can benefit your business.
2. Identify specific use cases where generative AI can add value to your business. This could include tasks such as content generation, creative design, product customization, and customer service automation.
3. Evaluate different generative AI tools and platforms available in the market to find the best fit for your business needs. Consider factors such as ease of integration, scalability, cost, and technical support.
4. Develop a clear roadmap for implementing generative AI in your business, including setting objectives, timelines, and KPIs to measure success. Ensure alignment with your overall business strategy and goals.
5. Collaborate with cross-functional teams within your organization to ensure a smooth integration of generative AI into existing workflows and processes. Provide training and support to employees to help them adapt to the new technology.
6. Continuously monitor and evaluate the performance of generative AI applications in your business, adjusting strategies and making improvements as needed. Stay informed about new advancements in AI technology to stay competitive in the market.
By following these steps and staying committed to innovation and learning, you can unlock the full potential of generative AI for your business and drive growth and success in the digital age.
FAQ: Getting Started with Generative AI
1. How can I get started with generative AI?
To get started with Generative AI, you can begin by familiarizing yourself with the basic concepts of artificial intelligence (AI) and machine learning. Understanding how generative models work and exploring tools designed for generative AI Introduction to generative AI workshops can help kickstart your learning journey.
2. What is an AI model in the context of generative AI?
An AI model in the context of generative AI refers to a computational system that is designed to generate new data based on patterns it has learned from existing data. These models are commonly used for tasks such as image generation and text generation.
3. How can I use generative AI to create unique content?
You can utilize generative AI tools to create diverse and original content such as artwork, music, or written text. By providing a prompt to the AI model, you can generate new outputs that can inspire creativity and innovation.
4. What are the potential use cases of generative AI?
Generative AI finds applications in various fields including content creation, design, healthcare, and more. It can be used for tasks like creating personalized recommendations, generating realistic images, or enhancing natural language understanding.
5. How do generative AI models differ from traditional machine learning models?
Generative AI models focus on generating new data points that resemble the training data, whereas traditional machine learning models are typically used for classification or regression tasks. Generative models are particularly adept at capturing the underlying distribution of the data.
Glossary: Getting Started with Generative AI
- AI (Artificial Intelligence): Technology that enables machines to mimic human intelligence, including learning, reasoning, and self-correction.
- Generative AI: A type of AI that generates new, previously unseen content or data by learning from a dataset.
- Machine Learning: A subset of AI where machines learn and adapt from data without being explicitly programmed.
- Deep Learning: A type of machine learning that uses neural networks with many layers to learn from data.
- Neural Networks: Computing systems vaguely inspired by the human brain that can learn and make decisions.
- GANs (Generative Adversarial Networks): A class of AI algorithms used in unsupervised machine learning, implemented by a system of two neural networks contesting with each other.
- Natural Language Processing (NLP): The ability of computers to understand, interpret, and generate human language.
- Prompt Engineering: The process of designing inputs to an AI to elicit the desired outputs.
- SEO (Search Engine Optimization): The practice of increasing the quantity and quality of traffic to your website through organic search engine results.
- Parameters: Variables that the AI model uses to make its decisions and adjustments during learning.