The manufacturing industry is undergoing a significant transformation thanks to the revolutionary capacities of artificial intelligence. Leading manufacturing companies are utilizing AI to improve efficiency, precision, and productivity in various operations. AI’s integration in manufacturing covers a broad spectrum of applications, including predictive maintenance, optimizing supply chains, ensuring quality control, and forecasting demand. This innovative approach provides a fresh perspective on conventional processes. For manufacturers, adopting AI at present is a strategic decision that leads to operational modernization and a competitive edge in the industry.
The Impact of AI on the Manufacturing Industry
Artificial intelligence (AI) plays a crucial role in enhancing productivity, efficiency, and decision-making processes within the manufacturing sector. In production, AI-driven predictive maintenance is utilized to optimize maintenance schedules and reduce downtime by analyzing equipment data to predict potential issues. Machine learning algorithms improve supply chain management by estimating demand, managing inventory, and streamlining logistics operations. Robotics integrated with AI technology automate assembly lines, boosting precision and speed while adapting to changing production needs. AI-powered quality control systems accurately detect defects, ensuring uniformity in the final products. Moreover, AI is employed in smart manufacturing to monitor processes in real time and make prompt adjustments to maximize efficiency and minimize waste.
In general, AI transforms the manufacturing sector by promoting innovation, reducing costs, and enhancing operational efficiency. To gain a deeper insight into the significance of AI in manufacturing, let’s explore some common applications with real-world illustrations.
The Impact of Artificial Intelligence on the Manufacturing Industry – Use Cases and Examples
The incorporation of artificial intelligence (AI) in the manufacturing sector is leading to a significant transformation, pushing the industry towards remarkable progress and increased efficiency. Below are prominent AI applications in manufacturing, along with real-world illustrations.
Enhanced Market Insights with AI
Businesses can benefit from enhanced market insights using generative AI, which enables advanced data analysis for understanding consumer behavior and competitive environments. This approach accelerates market research, improves market segmentation, and allows for the exploration of new markets through synthetic data generation. By employing AI tools for continuous monitoring, predictive analysis, and strategic decision-making, companies can stay ahead in the competitive landscape.
- Generative AI enhances market intelligence by automating data processing from various sources like social media and customer feedback.
- Benefits include accelerated market research, improved market segmentation, and continuous monitoring of competitors and market shifts.
- AI tools can be used for predictive analysis, synthetic data generation, and dynamic visualization of market insights.
- Businesses can leverage AI-generated insights for decision-making across marketing, product development, and sales strategies.
- Overall, generative AI offers timely and actionable insights, improves understanding of consumer behavior, and supports proactive business planning.
Cobots
Cobots, collaborative robots working alongside human operators, are revolutionizing AI-driven manufacturing by boosting productivity and efficiency in tasks like picking, packing, and quality checks. Companies like Amazon leverage cobots equipped with machine learning to accelerate order processing, streamline logistics, and enhance operational workflows. These intelligent robots excel in precise assembly tasks, quality control, and maintenance, showcasing a forward-thinking approach to industry productivity through human-machine collaboration.
- Cobots optimize fulfillment center workflows by enhancing picking, packing, and quality check efficiency.
- Tailored machine learning algorithms improve cobot recognition and handling capabilities.
- Real-time inventory updates reduce errors and enhance order accuracy.
- Continuous performance monitoring and data analytics refine operations for increased throughput.
- Pilot programs for new cobot applications scale productivity benefits across operations.
- Collaboration with cobot vendors ensures custom solutions tailored to unique challenges.
- A feedback loop with frontline workers enhances cobot integration and identifies areas for improvement.
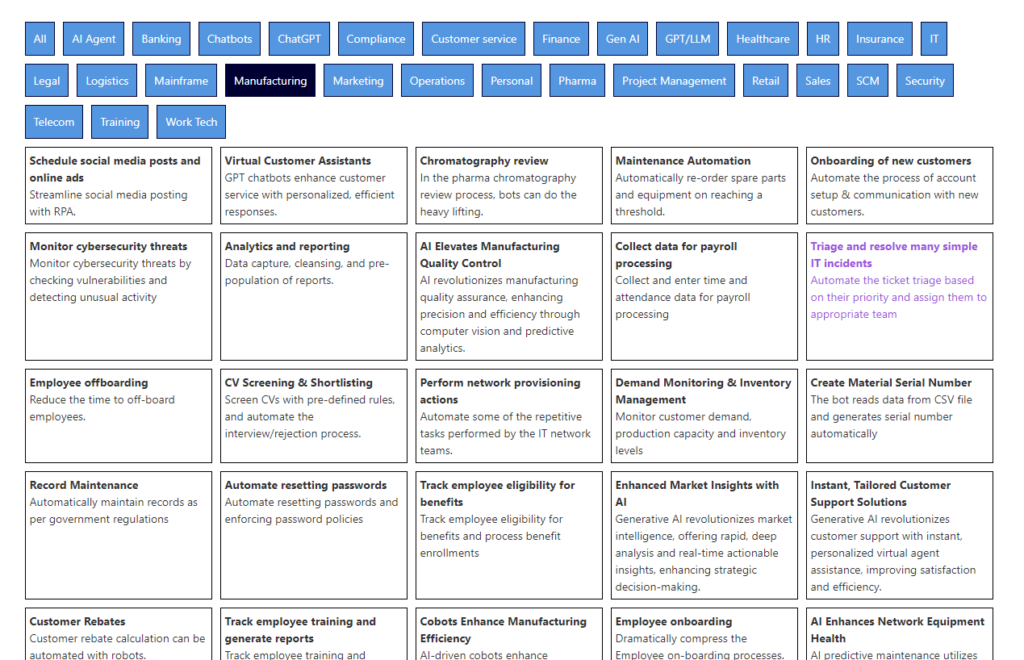
Assembly Line Optimization
AI-driven optimization in assembly line operations revolutionizes the manufacturing industry by enhancing precision, efficiency, and adaptability. Through the application of predictive maintenance, machine learning algorithms, and computer vision systems, companies like Volkswagen have exemplified the benefits of integrating AI solutions into their production processes. By analyzing real-time data, anticipating maintenance needs, adjusting workflows dynamically, and ensuring product quality, AI enables continuous improvement, waste reduction, enhanced resource utilization, and sustained manufacturing excellence.
- Implement AI-driven predictive maintenance to reduce downtime and enhance production efficiency.
- Utilize machine learning algorithms for real-time analysis of sensor data to optimize workflows.
- Integrate computer vision systems for quality inspection and defect detection.
- Adapt production parameters based on demand fluctuations for optimal resource utilization.
- Continuously analyze historical performance data for refining assembly line operations.
- Collaborate with AI solution providers to tailor applications to specific manufacturing challenges.
- Train teams on AI tools to foster innovation and continuous improvement.
- Monitor AI performance regularly for maximum efficiency and output.
- Ensure ethical considerations and compliance in AI applications.
- Stay informed on the latest AI advancements through industry engagement and forums.
Predictive maintenance
Predictive maintenance, powered by artificial intelligence, has revolutionized the manufacturing sector by enhancing equipment longevity and reducing operational costs. By leveraging predictive analytics and machine learning algorithms, AI-driven predictive maintenance enables real-time monitoring of equipment health, anticipating failures, optimizing maintenance schedules, and identifying areas for improvement. An exemplary application of this technology is Ford’s use of digital twin technology to proactively address energy losses and performance bottlenecks in its manufacturing processes. Through the integration of digital twins and sensor data, AI predicts and mitigates equipment failures, significantly improving operational reliability and efficiency in the manufacturing industry.
- Deploy sensors on critical equipment for real-time data collection and analysis.
- Integrate digital twin technology to simulate equipment behavior and identify potential failure points.
- Implement machine learning algorithms to predict failures and optimize maintenance schedules.
- Establish a centralized AI platform for comprehensive predictive maintenance analysis.
- Train AI models with vast datasets to enhance accuracy in predicting equipment failures.
- Conduct pilot projects to validate the effectiveness of AI predictive maintenance before full-scale deployment.
Track safety incidents and generate reports
RPA can be utilized to automate the collection and input of safety-related data from various sources like incident reports, safety inspections, and near-miss reports. The gathered data can be used to automatically generate reports on store safety performance, monitor key safety metrics and alert management about deviations. This proactive approach facilitates early issue identification, corrective actions, and enhancement of safety performance.
- RPA can automate data collection and generation of safety performance reports.
- Bots can monitor key safety metrics such as accident rate and near-miss rate.
- Alerts can be triggered for deviations from established norms, prompting remedial actions.
- Early identification of potential issues through automated monitoring helps in reducing accidents and injuries.
- The generated reports can aid in pinpointing areas for improvement and targeting specific safety training efforts.
Accelerating Product Design Innovation
Generative AI transforms product design by swiftly generating, refining, and simulating concepts, cutting development time and enhancing efficiency. It optimizes designs for performance and manufacturability, enables seamless team collaboration, and reduces the need for physical prototypes. This results in innovative, cost-effective products with faster market entry.
- Generative AI revolutionizes product design by swiftly generating diverse concepts, optimizing designs for performance and manufacturability, and facilitating real-time collaboration among teams.
- The integration of AI tools enables rapid concept generation, virtual prototyping, stress analysis, and seamless iteration of designs within existing CAD software.
- By simulating real-world performance early in the design phase, AI helps identify potential issues, reduce costly redesigns, and drive innovation to meet market trends effectively.
- The use of generative AI results in accelerated ideation, optimized designs, enhanced team collaboration, rapid prototyping with virtual models, and significant cost and time savings in product development.
- Continuous learning and adaptation of AI models through real-world performance data feedback ensure improved future design predictions and innovative product offerings to gain a competitive edge in the market.
Supply Chain Management
Supply chain management in the manufacturing industry is transforming with the adoption of artificial intelligence, leading to increased efficiency, accuracy, and cost-effectiveness. AI solutions facilitate predictive analytics, optimized inventory management, improved demand forecasting, and streamlined logistics processes. Walmart serves as a notable example of effectively utilizing AI in supply chain operations to enhance productivity and customer satisfaction. By employing AI for demand forecasting, inventory optimization, and logistics management, Walmart ensures product availability, minimizes stockouts and improves delivery speed. The incorporation of AI technologies into supply chain operations not only enhances decision-making and responsiveness but also boosts overall resilience in fiercely competitive markets.
- AI optimizes distribution and logistics in manufacturing by analyzing historical data to forecast demand accurately.
- Machine learning algorithms help coordinate distribution channels effectively by continuously assessing real-time variables like weather conditions and traffic patterns.
- AI-driven systems aid in inventory management by anticipating demand fluctuations, minimizing excess stock, and preventing shortages through predictive analytics.
- Automation of routine decision-making processes by AI results in streamlined supply chains and efficient resource utilization.
- AI transforms manufacturing supply chains by providing data-driven insights, leading to increased efficiency, reduced costs, and improved overall performance.
Quality Assurance
In the realm of manufacturing, AI-driven quality assurance is revolutionizing traditional quality control methods by introducing computer vision algorithms that swiftly detect defects and anomalies with unparalleled accuracy. This proactive approach not only reduces error margins and speeds up inspection processes but also enables manufacturers to adapt to market demands and technological advancements effectively. By leveraging predictive analytics and real-time monitoring, AI systems enhance production efficiency, product quality, and overall operational excellence.
- AI significantly lowers the chances of human oversight, ensuring product consistency.
- Computer vision accelerates defect detection, enhancing response time during quality control processes.
- AI algorithms excel in identifying subtle defects, improving overall product quality.
- Machine learning forecasts potential quality issues, enabling proactive corrections.
- Swift defect identification streamlines production lines, reducing downtime and waste.
- AI’s quick defect detection aligns production with technological advancements and market standards effectively.
Frequently Asked Questions (FAQs)
What is the use case of artificial intelligence (AI) in the manufacturing industry?
Artificial intelligence, often referred to as AI, plays a crucial role in the manufacturing industry by enabling manufacturers to implement AI solutions for various processes such as generative AI, predictive maintenance, automation, optimization, quality control, and manufacturing operations.
How is AI used in the manufacturing process?
AI is integrated into the manufacturing process to create efficient systems, optimize production, predict and prevent downtime, enhance quality control, automate tasks, and improve overall manufacturing operations. Using machine learning algorithms and generative design, AI enhances productivity and innovation.
What are some common AI use cases in manufacturing?
Common AI use cases in manufacturing include predictive maintenance, automating processes, optimizing the supply chain, enhancing quality control, and revolutionizing manufacturing operations through automation. The application of AI is diverse and impactful in the manufacturing sector.
How does AI benefit the manufacturing industry?
AI and machine learning technologies offer numerous benefits to the manufacturing industry by enabling manufacturers to optimize production processes, reduce costs, enhance product quality, and streamline manufacturing operations. By using generative AI and AI algorithms, manufacturers can stay competitive in the market.
What role does AI play in predictive maintenance in manufacturing?
AI systems are utilized in manufacturing for predictive maintenance to forecast equipment failures, prevent unplanned downtime, and optimize maintenance schedules.